https://doi.org/10.55788/ad120c2e
The lack of symptoms in the early stage of gastric cancer leads to delayed clinical presentation and low overall survival rate. Invasive screening, such as oesophagogastric duodenoscopy, is effective in decreasing gastric cancer mortality, but adherence is low [1,2]. Thus, there is a need for minimal or non-invasive gastric cancer screening, for example with routine blood tests. Algorithms based on routine blood tests were previously developed for the prediction of (colorectal) cancer, though low sensitivity, specificity, and positive predictive value limited their use [3,4].
Mr Tsz Chun Bryan Wong (Hong Kong University of Science and Technology, Hong Kong) and colleagues developed a deep learning AI algorithm using data from routine blood tests from more than 190,000 individuals who were prescribed medications for dyspepsia in the period between 2004–2015 [5]. Of these individuals, 4,790 patients were diagnosed with gastric cancer. The blood tests contained 29 parameters, including complete blood counts, liver function parameters, renal function parameters, and clotting function parameters.
Data from 2004 to 2009 and from 2011 to 2014 were used as a training cohort and the remainder was used as a testing cohort. A ‘gastric cancer’ signature in the blood was generated using deep-learning AI algorithms. After training and testing, the signature was able to predict gastric cancer with high sensitivity (96%), high specificity (100%), high positive predictive value (99%), and high negative predictive value (100%). In 2 large validation cohorts (n= 24,610 and n=17,058) the signature proved to be highly accurate (see Figure).
Figure: Validation of machine learning model to detect gastric cancer signature in blood [5]
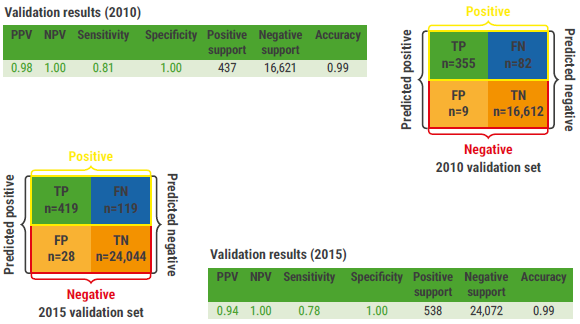
FN, false negative; FP, false positive; NPV, negative predictive value; PPV, positive predictive value; TN, true negative; TP, true positive
Based on these outcomes, Mr Wong concluded that “the blood signature can be used to enhance cost-effectiveness and public participation rates in screening for early gastric cancer.”
- Yashima K, et al. J Clin Med. 2022;11:4337.
- Suh YS, et al. Cancer 2020;126:1929–1939.
- Goshen R, et al. Br J Cancer. 2017;116: 944–950.
- Kinar Y, et al. J Am Med Inform Assoc. 2016;23:879–890.
- Wong TCB, et al. AI blood signature in common blood test for detection of gastric cancer in a cohort of 190,000 individuals. Abstract 1500, ASCO Annual Meeting 2023, 2–6 June, Chicago, USA.
Copyright ©2023 Medicom Medical Publishers
Posted on
Previous Article
« Response-directed treatment personalisation in stage III melanoma Next Article
No improved OS in pancreatic cancer after neoadjuvant mFOLFIRINOX »
« Response-directed treatment personalisation in stage III melanoma Next Article
No improved OS in pancreatic cancer after neoadjuvant mFOLFIRINOX »
Table of Contents: ASCO 2023
Featured articles
Real-world data support new SOC in patients with SCLC
What can real-world evidence teach us about atezolizumab plus bevacizumab in HCC?
Colorectal Cancer
7-year outcomes of PRODIGE 23 trial
Neoadjuvant chemotherapy may be viable option in locally advanced colon cancer
De-escalation of neoadjuvant treatment of locally advanced rectal cancer is non-inferior
Breast Cancer
SONIA: No survival benefit with first-line versus second-line CDK4/6 inhibition in metastatic breast cancer
Adjuvant ribociclib improves invasive DFS in early breast cancer
Gene expression profiles predict benefit of neoadjuvant immune checkpoint therapy in triple-negative breast cancer
Lung Cancer
Adding pembrolizumab to perioperative chemotherapy improves EFS in early-stage NSCLC
TTFields therapy: a new treatment modality for metastatic NSCLC
Adding chemotherapy to EGFR TKI does not improve OS in advanced EGFR-mutated NSCLC
Upper GI Cancer
No improved OS in pancreatic cancer after neoadjuvant mFOLFIRINOX
AI detects gastric cancer with high accuracy in common blood tests
Melanoma
Response-directed treatment personalisation in stage III melanoma
Prognostic and predictive biomarkers in patients with resected stage IIB/C melanoma
GU Cancers
Combining PARP inhibition and androgen receptor-signalling inhibition improves radiographic progression-free survival in HRR-deficient mCRPC
Erdafitinib outperforms chemotherapy in FGFR-altered advanced urothelial cancer
Probiotic CBM588 seems to improve clinical effect cabozantinib/nivolumab in mRCC
Exploratory analysis of IMvigor130 trial finds no OS benefit from atezolizumab in subgroups
Miscellaneous
Immune checkpoint inhibition improves PFS in non-BRCA-mutated ovarian cancer
First-line nivolumab-AVD improves PFS both in adult and paediatric patients with advanced Hodgkin lymphoma
Vorasidenib successfully targets IDH1/2-mutated glioma
ASCO Interviews
IMbrave050: Adjuvant atezolizumab plus bevacizumab provides landmark recurrence-free survival for HCC
What can real-world evidence teach us about atezolizumab plus bevacizumab in HCC?
Related Articles
September 17, 2020
Perioperative chemotherapy for resectable pancreatic ductal adenocarcinoma
June 25, 2019
Genomic and immune profiling
February 3, 2022
Support for fourth COVID-19 jab in blood-cancer patients
© 2024 Medicom Medical Publishers. All rights reserved. Terms and Conditions | Privacy Policy