https://doi.org/10.55788/368e63ab
A study by Dr Elizabeth Munroe (University of Michigan, MI, USA) aimed to assess risk-adjustment mortality models in patients with sepsis. “Traditional models do not fully incorporate acute physiology,” she outlined [1]. “Perhaps new machine learning models can do this better.” The authors used data from the HMS-sepsis registry, including 5,303 cases of sepsis hospitalisation in 31 hospitals across Michigan. The 90-day mortality rate of the sample was 27.0%. Several machine learning models were compared with a more traditional stepwise logistic regression model for the primary outcome of 90-day mortality. The models included patient characteristics, comorbidities, and parameters of acute physiology.
The stepwise logistic regression model had an area under the curve (AUC) of 0.77, whereas the best-performing machine learning model, Random Forest, had an AUC of 0.90. Dr Munroe explained that a disadvantage of the traditional model is its limited ability to assess interactions. In contrast, the more complex Random Forest model accounts for interactions. Furthermore, Radom Forest identified additional variables of importance for mortality in sepsis, such as creatinine and bilirubin levels, functional limitations, and dementia.
“Risk adjustment is important in sepsis quality care improvement,” according to Dr Munroe. “This study showed that machine learning models may help to improve risk adjustment in this population. A next step may be to use the variables that were identified by machine learning models to improve our traditional model.”
- Munroe E, et al. Machine learning methods to address confounding in sepsis mortality rate. C94, ATS International Conference 2023, 19–24 May, Washington DC, USA.
Copyright ©2023 Medicom Medical Publishers
Posted on
Previous Article
« Mitochondrial DNA levels predict COVID-19 severity Next Article
Efficient detection of AECOPD with at-home vital signs monitoring »
« Mitochondrial DNA levels predict COVID-19 severity Next Article
Efficient detection of AECOPD with at-home vital signs monitoring »
Table of Contents: ATS 2023
Featured articles
TORREY trial: seralutinib associated with reduced pulmonary vascular resistance in PAH
Practice-changing results for ensifentrine in COPD
Asthma
Vitamin D for asthma protection: what is the optimal timing?
Remarkable results for novel biologic therapy for asthma
Success for fluticasone furoate plus vilanterol in uncontrolled asthma
COPD
Practice-changing results for ensifentrine in COPD
Alvelestat for AATD meets primary endpoints in phase 2 trial
Fewer exacerbations with dupilumab in COPD with type 2 inflammation
New AATD therapy may alleviate treatment burden for patients
Efficient detection of AECOPD with at-home vital signs monitoring
COVID-19
COVA trial: success for the investigational agent sarconeos in hospitalised COVID-19
Mitochondrial DNA levels predict COVID-19 severity
Other
Excellent results for C21 in idiopathic pulmonary fibrosis
Can camlipixant improve quality of life of patients with refractory chronic cough?
TORREY trial: seralutinib associated with reduced pulmonary vascular resistance in PAH
Improving quality care in sepsis through machine learning models
Can information technology improve guideline adherence in sepsis care?
Related Articles
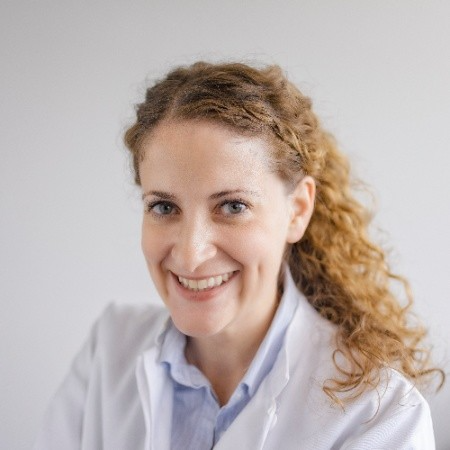
July 18, 2022
NAVIGATOR steers asthma patients to tezepelumab
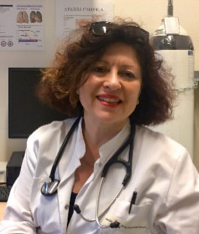
June 21, 2017
Letter from the Editor
© 2024 Medicom Medical Publishers. All rights reserved. Terms and Conditions | Privacy Policy